Penetration Test for AI?
No technology has quite had the impact on society and business than that of AI as of late. It's becoming increasingly hard to find a domain where AI isn't either actively used or at least being researched. Naturally, for us security people, AI is both a tool and a new type of threat landscape.
Let's cover the tool part first. Most security tools benefit from AI and machine learning. Whether it's detecting anomalies, identifying security issues or supporting security experts in filtering out the parts to focus on, AI shows great promise - if sometimes little concrete benefit for now - for virtually anything security.
For the threat landscape, a report titled "The Malicious Use of Artificial Intelligence: Forecasting, Prevention, and Mitigation" was released earlier this month. You can find it here.
It's a good overview of various types of threats - both those caused by AI and those facing AI-based implementations. If you have not gone through it, I highly recommend doing so if the topic is of interest. It's very understandable even if you are not too familiar with technical details of the field and does a good job at covering different angles of the same picture.
For a while now, I've been thinking about security threats facing AI implementations. Coming from the security background, one of the interesting questions for me has been, "what is the equivalent of a penetration test for AI?". See, in cybersecurity, penetration tests (or security tests or whatever you call them) are the de facto method that you generally apply to just about any kind of system - devices, web sites, hardware etc. Granted, security people might take offense in using the term so broadly but that's generally what our customers call it. It's a good catch-all term for the rough concept of using relevant testing methods to confirm if the level of security of the target is good or not. Customers use the term when they want to say "look this over, will you, and let me know if it looks good".
What, then, is the equivalent activity for AI?
Honestly, I don't quite know. Security testing of components in the system that uses AI is of course still perfectly valid and necessary approaches - AI didn't magically secure those parts. However, that is not strictly related to AI itself and is a bit of a cop-out. To attack it directly, we could look into few options.
We can make the AI make bad choices by...
- altering the training data so that the logic is faulty to begin with
- abusing the algorithm to force it to make undesirable decisions
Both of these are quite feasible options and there is plenty of literature on both approaches. Yet, I'm not quite sure what the AI penetration test would look like once (if) there will be a standardized business model around the topic. It also remains to be seen if that type of service is offered as an expert-driven activity or by other AIs that might be more suited to the kind of number crunching that is required to get anywhere.
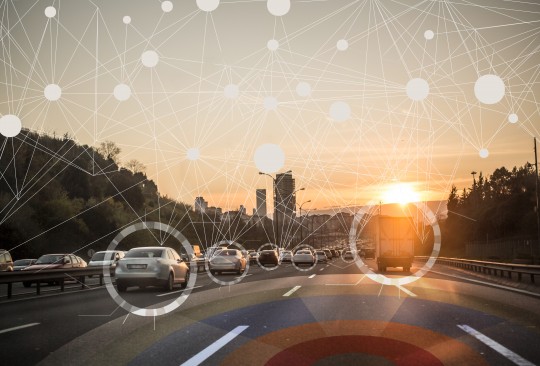
In some AI-driven domains, attacks and thus testing methods are quite easy to visualize. Imagine autonomous vehicles - what happens if we e.g. stand next to the road and hold up something that looks like a road sign? In such scenario, it's easy to poison the input data (= camera feed) coming to the car as the area around the road is untrusted and easy to manipulate. A human driver can likely spot and identify a rogue sign that makes no sense. For an AI, it might be more tricky as it doesn't really think - it merely tries to look for signs that help it to navigate and uses this information to find the best route. Who's to say that there can't be a sign here if you don't know any better.
In the end, I wonder if the next generation of penetration testing has me standing at the side of the road, dressed up as a carefully crafted "turn right here" sign in hopes of fooling the AI to take a sharp right turn.
Or, if we are going to see standardization in technologies that leads to standardization in attack approaches - just like how testing of web sites standardized into what it is today. In such case, we could see a standardized set of tools and methods that can attack poor designed or trained AI under specific conditions.
Either way, it's going to be a wild ride. Buckle up.
ps. I'm looking to follow up with a more in-depth discussion on AI-related threat landscape and what it means for cybersecurity. With any luck, I can convince some of my colleagues to join in on that and expand the topic more.